At a time when the issues surrounding the reindustrialization are at the heart of the economic and social agenda, it is essential to identify the levers for increasing the productivity of our industries.
In this respect, software innovation must be seen as a strategic area for modernization, in the same way as robotics. Among these software innovations, those relating to Computer Vision present concrete opportunities for boosting competitiveness!
What is Computer Vision?
Computer Vision is a scientific field that brings together all the techniques for capturing, processing, analyzing and measuring images. These methods enable computers to gain high-level understanding from digital images, videos and 3D models.
These techniques start with the mastery of hardware technologies for capturing digital images of various kinds: microscopic images (optical microscopes, electronic scanning microscopes), macro images (industrial cameras, cell phones), in 2D or 3D (scanners).
Image processing software solutions then come into play to exploit the digital data thus captured. They enable us to respond to the specific needs of our design offices and industrial production lines.
These image analysis solutions are based as much on historical techniques such as mathematical morphology, as on the latest generation algorithms such as Deep Learning :
- Mathematical morphology methods involve applying filters, also known as convolution masks, to a binary image to transform it. The transformations, which can be linear or non-linear, can be used to solve simple computer vision problems: identifying an object, segmenting an outline…
- Deep Learning is a sub-domain of Machine Learning, one of the techniques of artificial intelligence. It involves stacking “neural networks” to solve more complex problems, or to improve the performance of traditional algorithms.
The most robust and accurate computer vision solutions are often based on the combined use of morphological calculations and neural networks.
While the latter can produce more powerful results, they need to be trained on an annotated learning base. Let’s take a concrete example: the identification of defects in a cardan shaft production line for the automotive industry. For the Deep Learning algorithm to be effective, an expert will have to manually annotate hundreds or even thousands of cardan shafts from the production line to build up a high-quality learning base. Depending on the time required for this operation, the degree of expertise required and the availability of a team of experts, building up the learning base can be costly.
However, more conventional image processing methods can identify these defects with a certain degree of accuracy. They can be used to automatically pre-annotate a learning base. All that’s left for the expert to do is to validate the results (in the majority of cases) and correct them (in a small number of cases). So it’s the combined use of the two approaches that proves most effective!
All the more so as, to achieve the best results, Deep Learning techniques often require image pre-processing (contrast, filter, brightness, cropping) that falls within the scope of conventional image processing.
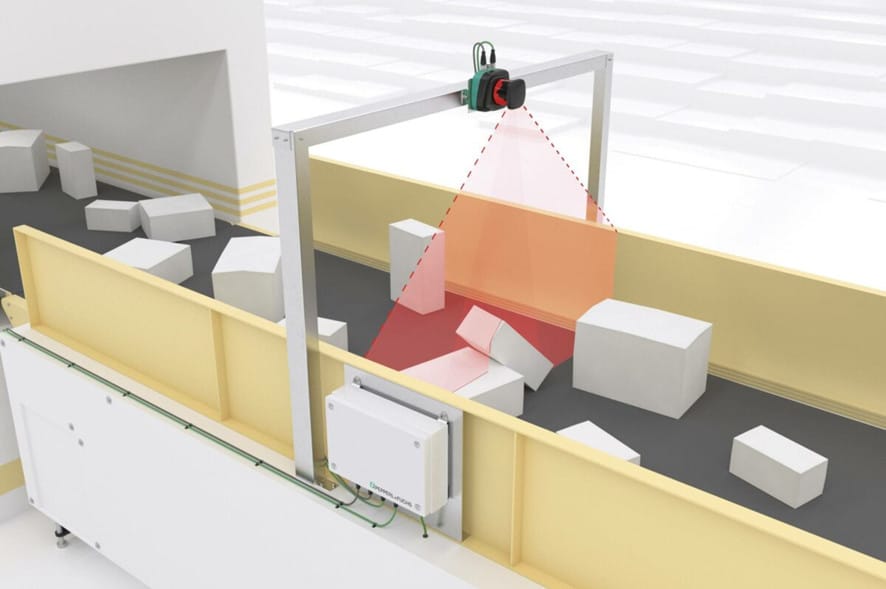
How does computer vision contribute to the competitiveness of our industries?
Computer Vision’s solutions enable us to automate a large number of tasks on our industrial production lines:
- Identify the position, speed and rotation of a set of parts in a flow.
- Count, measure, classify and segment objects in real time
- Characterize the matter and texture of materials
- Trigger processes or alerts
- …
Automation offers the advantages of greater traceability, and constant performance, i.e. not subject to concentration or availability problems. This performance is also scalable: it can be duplicated or its processing speed increased simply by adding computing power to the software.
Let’s take the example of the production of cardan shafts for the automotive industry. The cardan shafts that run along the high-speed production conveyor belt have to be inspected manually by expert operators. A well-trained artificial intelligence system classifies these parts into those of good quality, those which are faulty and therefore need to be isolated, and those for which the model has doubts and which need to be checked manually. This solution enables real-time analysis at high speed. The agent’s expertise is put to good use in the analysis of contentious cases. Another advantage of this system is that it can be configured to be self-learning: the expert’s decisions can be fed back into the learning base to improve its performance in terms of sensitivity and specificity (minimizing the number of false positives and false negatives).
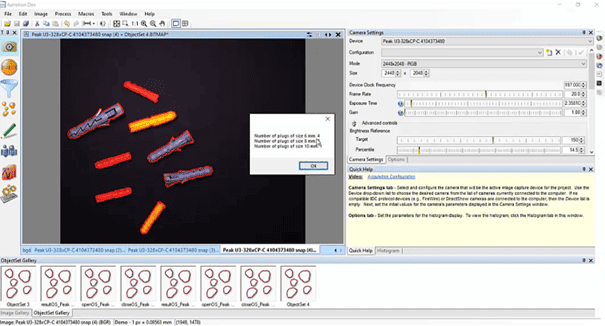
It’s easy to see how the automation of industrial tasks by “business” Computer Vision solutions offers numerous advantages in a number of concrete use cases:
- Product quality control (e.g. detection of defects in raw materials and manufactured products)
- Production line operation control (e.g. system alert generation, production interruption)
- Task automation (e.g. sorting, positioning, assembly)
- Preventive maintenance (e.g. wear detection, anticipation of risk factors)
- Product traceability throughout the chain
- Inventory management
Computer Vision is therefore a significant competitive lever for our production lines:
- Increased efficiency and speed of execution,
- Improving the quality of manufactured products,
- Reduced risk of errors and industrial accidents,
- Savings on raw materials,
- Personnel safety
The advantages are numerous and strategic. They have now been proven by many manufacturers who have chosen computer vision to transform their business models. While leading-edge industry players have already integrated computer vision into their industrial processes, decision-makers in more traditional industries still need to be made aware of the opportunities it opens up for them!